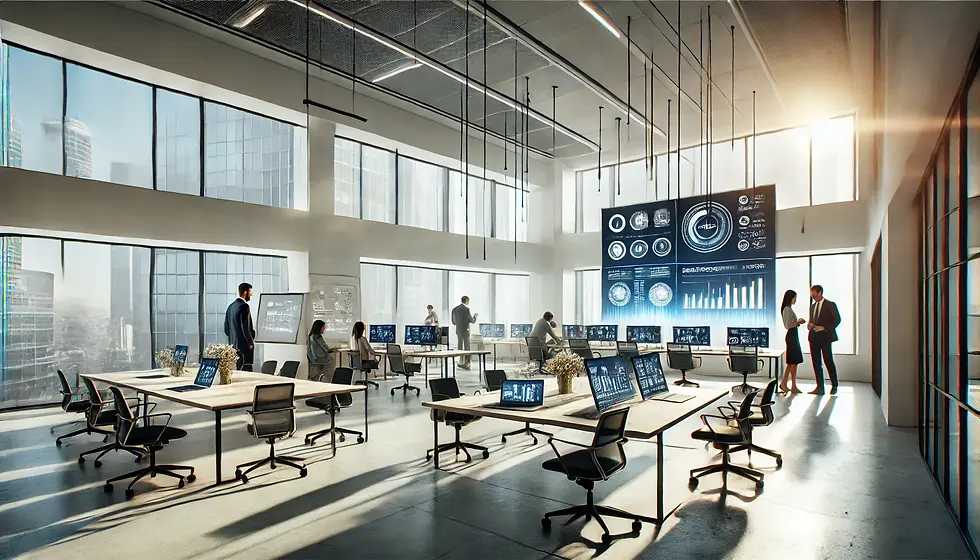
Successfully deploying AI in today’s competitive market is not simply about adopting the latest technologies. The real advantage lies in strategically aligning AI initiatives with core business objectives, prioritizing high-quality data, and integrating a team with the right expertise. As highlighted by Roger W. Hoerl and Thomas C. Redman in a recent MIT Sloan Management Review article, the effectiveness of AI depends on how well business leaders address these factors—especially by asking critical questions that go beyond the technical to emphasize strategic insights and practical applications.
Key Elements for AI Success
The Right Data: Quality Over Quantity
High-quality data is the cornerstone of reliable AI. However, not all data is created equal, and building robust AI models requires more than large datasets—it demands data that genuinely represents the complexity of the real world. AI models trained on narrow or unrepresentative data often underperform in real-world applications, leading to costly failures and inaccurate predictions. Business leaders should invest time and resources into curating data that is relevant, clean, and representative of the diverse scenarios the AI might encounter in its deployment. This “right data” provides a stable foundation for models, enabling them to generalize beyond controlled environments and consistently deliver valuable insights across varied situations.
Asking Strategic Questions: The Power of Inquiry in AI
While AI is inherently technical, its success in business settings is often a result of non-technical insights. Business leaders play a critical role in guiding AI by asking strategic questions during model development and deployment. For instance, managers should evaluate how the AI model’s outputs will be used, how it manages unexpected inputs, and whether it can reliably generalize beyond its initial training. These inquiries allow leaders to preempt potential issues—such as biased outputs or erratic behavior in novel situations—by ensuring the model is built with a clear understanding of its business context. This strategic questioning not only minimizes risk but also aligns AI efforts with broader organizational goals, enhancing the overall reliability and effectiveness of the AI system.
Assembling the Right Team: Multidisciplinary Perspectives Matter
AI development requires more than data scientists; it benefits from a diverse, multidisciplinary team that brings varied perspectives to the table. Statisticians, for instance, are instrumental in validating data quality and understanding variability, which is essential for creating models that perform under a wide range of conditions. Domain experts provide insights that ensure the model’s relevance and applicability in its specific industry, while end-users can offer practical feedback on usability and functionality. This cross-functional collaboration allows for a more comprehensive approach to AI development, reducing the risk of blind spots and making the technology more adaptable and reliable in its real-world applications.
Building Organizational Capabilities: A Culture of AI Readiness
To deploy AI successfully, businesses must also look inward and build organizational capabilities that support AI at scale. This includes investing in employee upskilling, fostering data literacy across teams, and promoting an understanding of AI’s limitations and ethical considerations. Educating business units on these factors not only leads to better decision-making but also mitigates the risks associated with over-reliance on automated processes or insufficient oversight. Organizations with a strong culture of AI literacy are better equipped to manage AI responsibly and to adapt as AI technology and applications evolve.
Aligning AI with Long-Term Goals
By prioritizing data quality, asking strategic questions, and fostering cross-functional collaboration, organizations can navigate the complexities of AI deployment more effectively. This approach enhances model performance and reliability and ensures that AI initiatives are aligned with long-term business goals. Such alignment not only reduces post-deployment setbacks but also fosters trust in AI systems among employees, customers, and stakeholders, laying the groundwork for sustainable success in AI-driven innovation.
Deploying AI effectively is a journey, one that requires continuous evaluation, collaboration, and education across the organization. With the right foundation, AI can transform businesses, driving growth and delivering value in ways that align with the evolving goals of the organization. By following these principles, business leaders can turn AI from a technical experiment into a strategic asset, fueling innovation and building a competitive edge.
#AIDeployment, #DataQuality, #BusinessStrategy, #AIinBusiness, #TechInnovation, #MachineLearning, #DataDriven, #AIImplementation, #DigitalTransformation, #AILeadership
Comments